Paul Grosu
Specializations: Bioinformatics • Genomics • Machine Learning
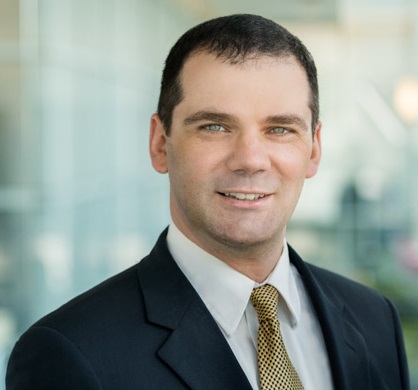
Throughout my career I was exposed to a variety of interesting problems. Some were hard and complex, which I enjoyed the most. I also enjoy synthesizing the complexities of such problems to make them understandable for a variety of audiences - as you have seen through my numerous GitHub posts. I enjoy merging together ideas across varied fields, such as distributed computer science, with complex data analysis for applications in the -omics domains.
For example, through the in-depth code and model analysis of Google’s DeepVariant, I am now able to adapt it towards many other integrated knowledge-discovery scenarios, for expanding either engineering-focused and/or science-focused opportunities.
An example of an engineering approach would be expanding implementation of DeepVariant via shared memory caches to maximize zero-copy optimizations. These could be further optimized by having such caches shared with GPUs for specialized parallelism. These serializations can be auto-traced for real-time temporal debugging, while validated via dynamic model checking contracts.
An example of a science approach would be to have automatic dynamic tensor constructions supervised under reinforcement learning. These machine learning approaches would explore optimal tensor designs that would best capture the data optimally for modeling additional characteristics about the genomics data. For example, if an ensemble set of models can capture different specialized sample characteristics across their molecular evolution, then somatic and clonal models can augment the germline ones. Thus new data can be checked across multiple models to see which models best respond, uncovering unknown sample characteristics for further investigation.
Another example of a science-based approach would be to generate analysis/classifier models for augmenting VCF files where the tuple <position, alleles, genotypes> would become query terms for augmentation. Such models can be truth-set supported knowledge-bases for labeling regions of similarity/dissimilarity as areas of interest for downstream analysis. Such labels could be disease-focused, pathways, or user-defined ones that can be loaded into a genome browser to highlight clusters of common labels. Within such clusters of interest, links to other public or community-shared datasets (or saved analysis) enable further validation expanding downstream analysis possibilities, and/or connect users with similarly labeled allele sets. This would be like a “VCFs + terms” query for a genomic Google search.
latest posts
Nov 14, 2023 | ShallowConsensus |
---|---|
Nov 4, 2023 | ShallowVariant |
Oct 18, 2023 | What do models learn? (Part 1) |